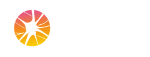
Sano – Centre for Computational Personalised Medicine
- International Research Foundation
Sano - Centrum Zindywidualizowanej Medycyny Obliczeniowej
– Międzynarodowa Fundacja Badawcza
Join to our newsletter
Copyright © 2023 Sano. All rights reserved. | Privacy policy | Selected images copyright OneHD/FNP.